Feeling the heat about proving your impact? Here’s a tool for reviewing all of the possible ways of measuring your initiative, organization or policy’s impact
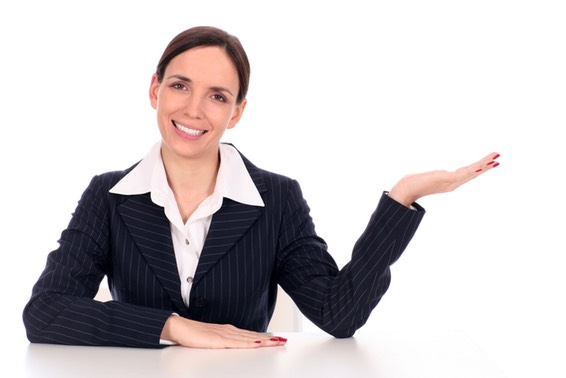
These days you are expected to measure your impact. But doing this can involve some technical work. To figure out how to do it, you first need to know the possible impact evaluation design types you could potentially use. Duignan’s Impact Evaluation Feasibility Check (Duignan 2009) is an easy way to navigate through the technicalities of impact measurement.
The tool identifies seven major impact evaluation design types. When you are trying to measure the impact of any project, program, initiative, organization or policy, you use the tool to systematically consider each of these seven possible impact designs. For each design type, you look at its potential appropriateness, feasibility, affordability and credibility in relation to your particular initiative.
Once you have done this, you can then assure yourself and, just as importantly your stakeholders, that you have exhaustively explored the potential ways of measuring your impact. You can’t promise your stakeholders that you will do the impossible in regard to impact measurement. However you need to be able to show them that you have left no stone unturned in examining what impact measurement might be appropriate, feasible, affordable and credible.
Some impact designs are highly technical, some less so
Some impact evaluation designs are relatively easy to use, for example Impact evaluation design type 6: Key informant judgment designs. In these designs, you simply ask people who are likely to be knowledgeable about the initiative or organization if they think that it has had an impact on outcomes.
However, other design types such as Randomized experimental designs and Time series designs are usually much more technical and will often will require that you involve someone with technical impact evaluation skills if you do not have these yourself.
The seven major impact evaluation design types used in the tool are listed below. A description is given of each of them. The idea is that for any initiative or organization you look through each of the design types and see if any of them are applicable in the case of your particular initiative or organization. At the begining of this process you cannot assume that any of these design types is necessarily going to be appropriate, feasible, affordable or credible for your particular initiative or organization until you have examined each of them in turn.
1. Randomized experiments and related designs
In randomized experimental designs, units (people, organizations etc.) are allocated randomly to an intervention and a control group. These are also called Randomized Controlled Trials (RCTs). Because random allocation has been used, all other confounding factors that may have produced any observed positive outcomes are ruled out as unlikely and the intervention is accepted as having had an impact.Related designs are regression discontinuity designs where units are not allocated randomly but on the basis of some other characteristic. For instance, units might be ranked from the worst to the best performers on an outcome measure measured before the initiative is run. The intervention is then only given to those below a cut-off score. If the intervention works you would expect to see an improvement in the intervention group relative to how they should have been expected to have performed based on the outcomes for the non-intervention group. (Regression discontinuity designs are included with experimental designs because they involve an experimental manipulation, however their rationale is similar to the more passive versions of such designs set out under the Constructed matched comparision group designs).
2. Time series designs (multiple observations over time)
In time series designs, a number of measurements of an outcome are taken over a substantial period of time. An intervention is then introduced at a specific point in time. If it is successful, it is expected that there would be an improvement at the time when the intervention was introduced (or after a known time lag).Because a series of measurements have been taken over time, it is possible to look at the point in time when the intervention was introduced and ask the question as to whether an improvement is shown at precisely that point.
For instance, an improvement can be seen visually by looking at a graph of the outcomes measured over time. More sophisticated versions of these designs can involve starting and stopping an intervention and then looking for effects at the particular points in time when the intervention was started and stopped.
4. Exhaustive causal identification and elimination designs
In exhaustive alternative causal identification and elimination designs there needs to be a good way of measuring whether or not outcomes have occurred. Then, if positive outcomes have been observed, all of the alternative explanations as to why these positive outcomes might have occurred are detailed.Each of the alternative explanations are then eliminated by logical analysis, and using any empirical data available. If all alternative explanations can be eliminated, it leaves the intervention as the only credible explanation as to why outcomes have improved. It is then just assumed that the intervention did actually cause the positive outcomes to occur.
These impact evaluation designs are sometimes seen as less robust and credible by some stakeholders than the designs listed above. However they are usually much more feasible and affordable.
3. Constructed matched comparison group designs
In constructed matched comparison group designs, the attempt is made to identify or create a comparison group which does not receive an intervention. This group is then used to compare outcomes against the group which does receive the intervention.For instance, one might find a similar community with which one could compare an intervention community.
In some cases, called propensity matching, statistical methods are used to work out what 'is likely to have happened’ to outcomes for a particular type of person or unit if they did not receive the intervention. This is done by looking at the outcomes for many people or units which did not receive the intervention and using statistics to predict expected 'non-intervention' outcomes. This then gives you an average estimate of what is likely to happen to people or units that do not receive the intervention. You can then compare this ’normative' information with the outcomes of those who actually did receive the intervention.
There are a number of techniques that can be used within Constructed matched comparison group designs and these are set out in the table at the bottom of this page.
5. Expert judgment designs (just asking experts)
In expert judgment designs, an expert, or an expert panel, is asked to make a judgment as to whether, in their opinion (using whatever method they usually use in making such judgments) they believe that the program has had an effect on improving high-level outcomes.This type of evaluation design is sometimes called a 'connoisseur' evaluation design drawing on an analogy with connoisseur judges such as wine tasters. Obviously this type of design can only be used in cases where you can locate people who are experts in the type of initiative or organization being evaluated.
These impact evaluation designs are sometimes seen as less robust and credible by some stakeholders than the designs listed above. However they are usually much more feasible and affordable.
7. Intervention logic (program theory/theory of change) designs
In intervention logic designs, first you build a credible 'intervention logic' (theory of change/strategy model) for the initiative or organization. It sets out how it is believed that lower-level program activities will logically lead to higher-level outcomes (for instance, this can be done in the form of a visual DoView Strategy Model).You then validate this logic against previous evidence in similar cases or get experts in the topic to endorse it as a credible logic. It is then established that lower-level activities have actually occurred (relatively easy to do because they tend to be able to be measured by controllable, and therefore attributable, indicators). The final step in the process is to just assume (but know that you have not proved) that the lower-level activities did, in this particular instance cause higher-level outcomes to occur.
These types of impact evaluation designs are sometimes seen as less robust and credible by some stakeholders than the designs listed above. However they are usually much more feasible and affordable.
6. Key informant judgment designs (asking those who might know)
In key informant judgment designs, key informants (people who are likely to be in a position to be knowledgeable about what is happening in an initiative or organization and whether it impacted on high-level outcomes) are asked to make a judgment regarding whether they think that the program has actually affected high-level outcomes (using whatever method they want to use to make such judgments).These impact evaluation designs are sometimes seen as less robust and credible by some stakeholders than the designs listed above. However they are usually much more feasible and affordable.
An example of using Duignan’s Impact Evaluation Feasibility Check™
In practice you use Duignan’s Impact Evaluation Feasibility Check™ by going through each of the above seven impact evaluation design types and assessing the appropriateness, feasibility, affordability and credibility of each of them. A design's appropriateness looks at, for instance, whether there are ethical or political reasons why the design should not be used. Its feasibility looks at, in those instances where a design is potentially appropriate, whether there are technical problems with implementing the design. A design's affordability is the cost of doing the evaluation using the particular design. Its credibility is the extent to which stakeholders and evaluation audiences are likely to accept the findings of an evaluation.
Below is an example of how the tool can be applied. The example used is of a new building regulatory regime introduced as a consequence of the failure (due to leaking) of a number of buildings built under the previous building regulatory regime.
1. Randomized experiments and related designs
Randomized experimental designs
NOT CONSIDERED APPROPRIATE. Such designs would set up a comparison between a group which receives the intervention and a group (ideally randomly selected from the same pool) which does not. In the case of this regulatory intervention, for ethical, political, legal and design-compromise reasons it is not possible to implement this particular intervention in one or more localities while other localities (serving as control groups) do not receive the intervention. Apart from anything else, statutory regulation could not be imposed on only part of the country.
Secondly, there is a major impact evaluation design-compromise problem that could rise from a situation where there might be higher standard of new building work in one locality (the intevention locality) but not in another (the control locality). It is likely that compensatory rivalry could reduce any difference in outcomes between the intervention and control group.
Compensatory rivalry is where the control locality also implements an intervention similar to that which is being evaluated because it also wants to achieve the outcomes which are as important to it as they are to the intervention locality. This can happen when the control locality finds out about the details of the intervention being implemented in the intervention locality. It can then start to copy the control locality’s intervention. This makes it less likely that any effect will be found from the intervention. Since this type of design is not considered appropriate, its feasibility, affordability and credibility have not been assessed.
Regression discontinuity designs
NOT CONSIDERED APPROPRIATE. One example of a regression discontinuity design that could theoretically be used in this case would be if you graphed the localities that could potentially receive the intervention on a measurable continuum (e.g. the current quality of new buildings in the locality). The intervention would then only be applied to those localities below a certain cut-off level (i.e. those with the poorest quality buildings). The intervention (the new regulatory framework) would only be applied to those localities. Any effect should show up as an upwards shift of the graph of outcomes for intervention localities after the intervention has been run for a suitable period of time.
In theory, it might be possible to rank localities in order of the quality of their new building work. If resources for the intervention were limited, it would be ethical to only intervene in those localities with the worse new building work and hence use a regression discontinuity design. However, as discussed above for randomized experiments, in this case the political, legal and design-compromise issues mean that a regression-discontinuity design does not seem appropriate. Since this type of design is not considered appropriate, its feasibility, affordability and credibility have not been assessed.
2. Time-series designs
NOT CONSIDERED APPROPRIATE OR FEASIBLE. These designs measure outcomes a large number of times (say 30) before, during, and after an intervention has occurred. They then look to see if there is a clear change at the point in time when the intervention was introduced. These designs would be possible if multiple measurements of new building quality (the outcome) were available over a lengthy (say 20 year) period. Time series could then be used to track changes in the outcome over the course of the intervention. In the first instance, the length of time for this design to provide information makes it inappropriate even if it could be implemented.
However this design also has the design-compromise problem that there is another major factor potentially affecting outcomes. This can be termed the ‘crystallization of liability’ which is occuring at the same time as the introduction of the new building regulatory regime.
The crystallization of liability is a consequence of all of the relevant stakeholders now becoming aware of the liability they can be exposed to due to failure of many buildings and the attendant liability claims which have arisen for them. It should be noted that this crystallization, of course, does not mean that any available time series data cannot be used as a way of tracking improvements over time in the quality of new building work. It is just that any such time series analysis would be silent on the question of attribution of any improvement in building quality to the new building regulatory regime rather than to the crystallisation of liability being the factor that caused any observed improvement to occur. Since this type of design is not considered appropriate, its feasibility, affordability and credibility have not been assessed.
3. Constructed matched comparion group designs
APPROPRIATE BUT NOT CONSIDERED FEASIBLE. These designs would attempt to locate a group which is matched to the intervention group on all important variables apart from receiving the intervention. This is an appropriate design. It would require the construction (identification) of a comparison group not subject to a change in its building regulatory regime, ideally over the same time period as the intervention. Since the new building regulatory regime is a national intervention, such a comparison group would not be able to be located within the country in question. It is theoretically possible that one or more comparison groups could be constructed from other countries or regions within other countries.
However, discussions so far with experts in the area have concluded that it is virtually impossible for a country or region to be identified which could be used in a way that meets the assumptions of this design. These assumptions are: that the initial regulatory regime in the other country is the same; that the conditions new buildings are exposed to in the other country are similiar; that the authorities in the other country do not respond to new building quality issues by changing their regulatory regime themselves; and that there are sufficient valid and reliable ways of measuring new building quality in both countries before and after the intervention. It should be noted that while some of these assumptions may be met in regard to some overseas countries or regions, all of them would need to be met for a particular country or region to provide an appropriate comparison group within a constructed matched comparison group design. This design is appropriate but not feasible therefore its affordability and credibility have not been assessed.
4. Causal identification and elimination design
APPROPRIATE BUT NOT CONSIDERED CURRENTLY FEASIBLE BUT INVESTIGATE. The first step in this design is identifying that there has been a positive change in observed outcomes. The second step then identifies all of the possible alternative reasons for the improvement apart from the intervention being evaluated. Lastly, it rigorously analyses each possible alternative explanation and eliminates all of the alternative explanations. This leads to the conclusion that it is likely to be the intervention that has caused the observed improvement in outcomes.
This approach needs to go beyond just accumulating evidence as to why it may be possible to explain the observed outcome as resulting from the intervention. It requires that the alternative explanations be rigorously eliminated as being the cause of the outcome. This approach is sometimes described as a ‘forensic’ approach because it is similar to the way in which detectives work.
This is a potentially appropriate design in the case of introducing the new building regime. But in terms of feasibility, taking this approach may not be possible in this case. As with earlier designs, the concurrent crystallization of liability which is occurring in the same timeframe as the intervention is a problem. It is likely that this other potential cause of improvements in outcomes is significantly interwined with the intervention in being responsible for any improvement that occurs in the outcome of new building quality. It should be investigated whether it would be possible to untangle the effect of the intervention from the effect of crystallization. This will be discussed in the designs below. The affordability and credibility of this design has not been assessed.
5. Expert judgement design
POTENTIALLY HIGH APPROPRIATENESS, UNCERTAIN FEASIBILITY, HIGH AFFORDABILITY, LOWER CREDIBILITY. This design consists of asking subject matter expert(s) to analyze and assess in a way that makes sense to them whether, on balance, they accept the hypothesis that the intervention is likely to have caused observed positive outcomes. One or more well-regarded and appropriate independent expert(s) in building regulation (presumably from overseas in order to ensure independence) could potentially be asked to visit the country and to assess whether they believe that the new regulatory regime has led to some improvements in new building quality. This would be based on their professional judgment and they would take into account what data they believe they require in order to make their judgment. Their report would spell out the basis on which they made their judgment.
When this design is used the evaluation question being answered should always be clearly identified as: In the opinion of an independent expert(s) has the new building regulatory regime led to an improvement in building outcomes? The feasibility problem with this design is the same one as in regard to earlier designs - the crystallization of liability not being able to be untangled from the impact of the new building regime itself. This is discussed below.
6. Key informant designs
POTENTIALLY HIGH APPROPRIATENESS, UNCERTAIN FEASIBILITY, HIGH AFFORDABILITY, LOWER CREDIBILITY. A key informant judgment design consists of asking key informants about the impact of an initiative. Key informants are people who, because of their position, are likely to be know about what occurred in an intervention and its likely impact on outcomes. They are asked to analyze the success of the intervention in a way that makes sense to them and to assess whether, on balance, they accept the idea that the intervention may have caused the observed positive outcomes. A selection of stakeholder key informants could be interviewed face-to-face in regard to the new building regime and their opinions regarding what outcomes can be attributed to the new building regime could be summarized and analyzed in order to draw general conclusions about the effect of the intervention. This could be linked in with an expert judgment and causal elimination design as described above.
The crystallization of liability problem is also a problem here. One way to examine how much of a problem it is would be to interview or talk to a group of people involved in the building industry and put the problem to them. The key question is whether those involved in the industry would be able to tease out the two possible determinants of how they are behaving - being influenced by the new building regulatory regime or crystallization of liability. For instance, you can imagine a question such as: ‘in your day to day work are you more influenced by the fear of being liable for mistakes that are made or are you just worried that your work will not pass an inspection’.
7. Intervention logic design
CONSIDERED HIGH APPROPRIATENESS, HIGH FEASIBILITY, HIGH AFFORDABILITY, LOWER CREDIBILITY. An intervention logic design consists of three elements. The first is measuring whether or not high-level outcomes have improved. The second focuses on the intervention logic of the initiative. An intervention logic, theory of change or strategy model sets out the high-level outcomes for an initiative and the steps that it is believed will lead to these outcomes. The second step is, therefore, to show that the initiative’s intervention logic is evidence-based and robust. One way of doing this is to send it out for expert review by appropriately qualified and experienced experts. The third, and final, step in an intervention logic design is to confirm that the lower-levels of the intervention logic have actually been done by the initiative. If these three elements are all in place then this design assumes that it is the initiative that has caused the high-level outcomes to occur.
Use of the approach
Anyone can use the above material when doing evaluation planning for their own organization or for-profit or not-for-profit consulting work as long as they acknowledge that they are using Duignan’s Impact Evaluation Feasibility Check™ and include the reference to it below. If you would like to have us work with you applying this tool to an evaluation please get in ouch using the Duignan Evaluation Planning Clinic™ contact page.
You cannot embed this approach into software apps or web-based systems without our permission. If you want to embed it in such systems please contact use through the contact page on this site.
The reference that should be included when you are using this tool is: Duignan, P. (2009). A concise framework for thinking about the types of evidence provided by monitoring and evaluation. Australasian Evaluation Society International Conference, Canberra, Australia, 31 August - 4 September, 2009. For more information see Duignan’s Impact Evaluation Check™ http://parkerduignan.com/m/impact-evaluation.
We love getting feedback on our work. If you enjoyed using this tool please let us know and if you have any feedback for improving it we would love to hear it. Contact us here.
Details of the techniques that can be used in Constructed matched comparison group designs
Technique
What is possible in the situation
How to do it
Difference-in-difference
Instrumental variable
For instance, they may live a long way away from where the intervention is going to be held - so the reason they have not chosen to go into the intervention group is not because of something like motivation (which could be related to the outcome) but simply because of travel costs (which it is believed will be unrelated to the outcome).
This means that you can compare the results for the intervention group and this similar untreated comparison group as the only thing that is different between then is that the intervention group is receiving the intervention.
Propensity matching
For each of the members of the intervention group use the formula to predict what their outcomes would have been likely to be WITHOUT THE INTERVENTION. Compare their actual results on the outcomes (after they have received the intervention) with their predicted results (which are likely to have occurred if they had NOT received the intervention).
Case matching
FC2018